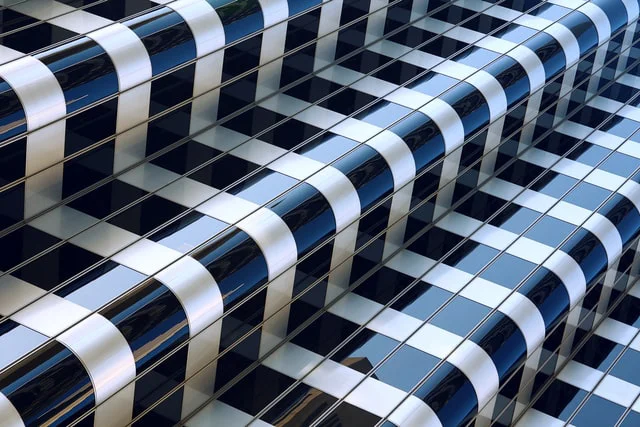
Cumulative standard normal distribution
July 07, 2019 3 minutes reading time Math go rust statistics
I’ve written about the use of the central limit theorem (CLT) to solve some problems in statistics before. It involves calculating a z-value and looking up the probability in a z-table. A z-table holds the cumulative probabilities for values that follow the standard normal distribution, i.e. the mean is 0 and the standard deviation is 1. For other means and standard deviations, the values can be normalized, so that mean/standard deviation of the standard normal distribution apply.
In programs like Excel or in code, we can easily calculate the z-table values. Some languages like Java (Apache Commons Math) or Rust (libm crate) require the use of 3rd party libraries if you don’t want to do all the work yourself, but Go has everything built-in already. Let’s see how to do it.
The cumulative distribution function for a function with normal distribution: $$\phi\left(x\right) = \frac{1}{2}\left(1+\textbf{erf}\left(\frac{x-\mu}{\sigma\sqrt{2}}\right)\right)$$
Where $\textbf{erf}$ is the error function: $$\textbf{erf}\left(z\right) = \frac{2}{\sqrt{\pi}}\int_0^z e^{-t^2} dt$$
Go has the error function $\textbf{erf}$ in the math
package, so it’s easy to write $\phi(x)$ as a higher-order-function, that takes the mean $\mu$ and the standard deviation $\sigma$ and gives us a function that only depends on the random variable x
.
With this we can write all the code necessary to solve the statistics problem mentioned above.
package main
import (
"fmt"
"math"
)
func phi(m float64, sd float64) func(float64) float64 {
return func(x float64) float64 {
z := (x - m) / sd / math.Sqrt(2)
return (1 + math.Erf(z)) / 2
}
}
func main() {
maxWeight, boxes, m, sd := 2630.0, 36.0, 72.0, 3.0
// the central limit theorem states that if we have a
// sufficiently large sample of n boxes, we can expect
// the mean of this sample to be equal to the statistical
// mean, and the σ_sample of the sample to be σ / sqrt(n)
zTable := phi(0, 1) // cumulative std. normal distribution
boxCritical := maxWeight / boxes
sdSample := sd / math.Sqrt(boxes)
// normalize for z-table use
z := (boxCritical - m) / sdSample
p := zTable(z)
// probability that boxes can be transported
fmt.Printf("%f\n", p)
}
Rust has only a minimal standard library by design, where the error function is not included. But you can use the libm crate for that.
use libm::erf;
fn phi(m: f64, sd: f64) -> impl Fn(f64) -> f64 {
move |x| {
(1.0 + erf((x - m) / sd / 2_f64.sqrt())) / 2.0
}
}
fn main() {
let max_weight : f64 = 2630.0; // in kg
let boxes : f64 = 36.0; // amount of boxes
let m : f64 = 72.0; // mean of the historic boxes
let sd : f64 = 3.0; // standard distribution of the historic boxes
let z_table = phi(0.0, 1.0); // cumulative std. normal distribution
let box_critical = max_weight / boxes;
let sd_sample = sd / boxes.sqrt();
// normalize for z-table use
let z = (box_critical - m) / sd_sample;
let p = z_table(z);
// probability that boxes can be transported
println!("{}", p);
}
We could have used the $\phi$ function without normalizing, since it can handle arbitrary means and standard deviations, but I wanted to show a way that mimics how you would do it by hand using a z-table.